Most proteins interact with each other and function in the form of complexes (1). Determinization of these protein-protein interactions (PPIs) in vivo thus plays an essential role in understanding their regulation and coordination (2). For different purposes, three DIA-MS implementations are commonly applied to PPI studies.
AP-DIA-MS Affinity purification (AP) coupled with MS (3) uses the proteins of interest as the “bait” and collects their binding partners (“preys”) for identification. In 2013, Lambert et al. and Collins et al. designed an AP-DIA-MS setting (2, 4), wherein DIA was realized with SWATH-MS, and manually analyzed the data with a library-based, peptide-centric approach. This method has been used to study interactome differences under internal (gene mutation) and external (drug treatment) perturbations, respectively (2). Remarkably, 1,967 proteins with a quantification dynamic range of over four orders of magnitude could be profiled in the temporal interactomes of 14-3-3β system (4). Later, AP-DIA-MS has seen updates from technical support (5) expansions to several biomedical applications, including targeting tumor markers (6) and determining drug affinity responsive and its target stability (7). However, AP-MS cannot be used when the targets are unknown.
XL-DIA-MS In cross-linking (XL)-MS, an analyte can either belong to the targeted protein complexes or the rest of the untargeted proteome. A chemical cross-linker is added to the analyte and covalently links adjacent amino acid residues before MS measurement (8). The topological information provided by XL-MS can be used to reconstruct the spatial structure of PPIs (9). Muller et al. benchmarked XL-DIA-MS with seven chemically cross-linked proteins (10) and found that DIA-MS provides higher accuracy in quantitation and better reproducibility than DDA-MS. Additionally, this method was further coupled with photoactivatable XL to study pH-dependent conformational changes of a protein complex (11). The main limitation of XL-DIA-MS is that the reported interactions are biased towards high abundant proteins, compromising the identification coverage (1).
SEC-DIA-MS Size exclusion chromatography (SEC) separates the analytes depending on their relative size and hydrodynamics (12). SEC-MS is thus an untargeted approach that preserves the native properties of system-wide protein complexes (13). Heusel et al. applied SWATH-MS to acquire interactomics information from HEK293 lysates (14). The algorithm CCprofiler was then developed to infer and quantify proteins and protein complexes. The algorithm for the latter process was termed “complex-centric” because, similarly to the peptide-centric data search, it also involves a targeted data extraction step to derive elution profiles of complex subunits based on an a priori PPI database. Results showed that 55% of the detected protein mass is in the form of protein complexes entailing 462 complexes and 2,127 protein subunits. An alternative approach termed “network-centric” evaluates the edges (relations) rather than the points (proteins) or the clusters (protein complexes) in the PPI network (15). The network-centric software SECAT was applied to compare PPI differences between cell cycle states and provided interactomics alterations with a higher resolution. Comparatively, the network-centric approach is better suited to study phenotype-associated molecular mechanisms (15, 16). In addition, the recent software PCprophet (17) enables de novo PPI-free protein complex predictions. Bearing high potential, over 1000 complexes composed of over 10,000 PPIs could be retrieved from the HeLa cell line.
Several alternative MS-based approaches are also popularly used to characterize PPIs and complex structures and can potentially be integrated with DIA-MS. For example, thermal proteome profiling measures PPI thermal stability and abundance (18), while MS footprinting uses hydrogel radiation reactions to characterize the PPI domains at different conditions (19). An additional potential of DIA-MS-based PPI studies might be the meta-analysis of full proteome co-variation, which can collect data to identify novel protein complexes and PPIs across species and individuals. As an initial test, Stalter et al. analyzed protein complex co-variations using the SWATH-MS profiles of four species (20). They found that homomeric paralogues display a unique co-expression pattern distribution.
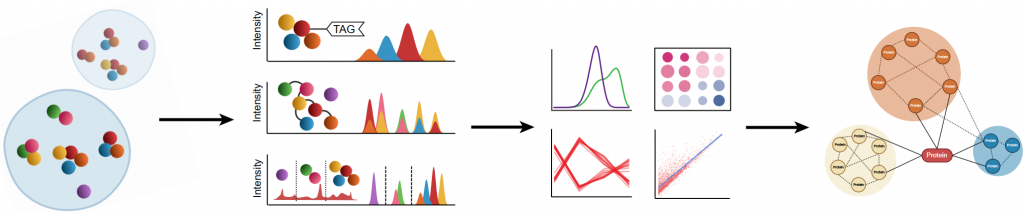
References
1. Bludau I. Discovery-Versus Hypothesis-Driven Detection of Protein-Protein Interactions and Complexes. Int J Mol Sci. 2021;22(9).
2. Lambert JP, Ivosev G, Couzens AL, Larsen B, Taipale M, Lin ZY, et al. Mapping differential interactomes by affinity purification coupled with data-independent mass spectrometry acquisition. Nat Methods. 2013;10(12):1239-45.
3. Ho Y, Gruhler A, Heilbut A, Bader GD, Moore L, Adams SL, et al. Systematic identification of protein complexes in Saccharomyces cerevisiae by mass spectrometry. Nature. 2002;415(6868):180-3.
4. Collins BC, Gillet LC, Rosenberger G, Röst HL, Vichalkovski A, Gstaiger M, et al. Quantifying protein interaction dynamics by SWATH mass spectrometry: application to the 14-3-3 system. Nat Methods. 2013;10(12):1246-53.
5. Liu G, Knight JD, Zhang JP, Tsou CC, Wang J, Lambert JP, et al. Data Independent Acquisition analysis in ProHits 4.0. J Proteomics. 2016;149:64-8.
6. Kobayashi D, Tokuda T, Sato K, Okanishi H, Nagayama M, Hirayama-Kurogi M, et al. Identification of a Specific Translational Machinery via TCTP-EF1A2 Interaction Regulating NF1-associated Tumor Growth by Affinity Purification and Data-independent Mass Spectrometry Acquisition (AP-DIA). Mol Cell Proteomics. 2019;18(2):245-62.
7. Kim D, Hwang HY, Kim JY, Lee JY, Yoo JS, Marko-Varga G, et al. FK506, an Immunosuppressive Drug, Induces Autophagy by Binding to the V-ATPase Catalytic Subunit A in Neuronal Cells. J Proteome Res. 2017;16(1):55-64.
8. Götze M, Iacobucci C, Ihling CH, Sinz A. A Simple Cross-Linking/Mass Spectrometry Workflow for Studying System-wide Protein Interactions. Anal Chem. 2019;91(15):10236-44.
9. Iacobucci C, Götze M, Sinz A. Cross-linking/mass spectrometry to get a closer view on protein interaction networks. Current Opinion in Biotechnology. 2020;63:48-53.
10. Zhang S, Di Y, Yao J, Wang Y, Shu H, Yan G, et al. Mass defect-based carbonyl activated tags (mdCATs) for multiplex data-independent acquisition proteome quantification. Chem Commun (Camb). 2021;57(6):737-40.
11. Müller F, Graziadei A, Rappsilber J. Quantitative Photo-crosslinking Mass Spectrometry Revealing Protein Structure Response to Environmental Changes. Analytical chemistry. 2019;91(14):9041-8.
12. Barth HG, Jackson C, Boyes BE. Size Exclusion Chromatography. Analytical Chemistry. 1994;66(12):595-620.
13. Kristensen AR, Gsponer J, Foster LJ. A high-throughput approach for measuring temporal changes in the interactome. Nat Methods. 2012;9(9):907-9.
14. Heusel M, Bludau I, Rosenberger G, Hafen R, Frank M, Banaei-Esfahani A, et al. Complex-centric proteome profiling by SEC-SWATH-MS. Mol Syst Biol. 2019;15(1):e8438.
15. Rosenberger G, Heusel M, Bludau I, Collins BC, Martelli C, Williams EG, et al. SECAT: Quantifying Protein Complex Dynamics across Cell States by Network-Centric Analysis of SEC-SWATH-MS Profiles. Cell Syst. 2020;11(6):589-607.e8.
16. Heusel M, Frank M, Köhler M, Amon S, Frommelt F, Rosenberger G, et al. A Global Screen for Assembly State Changes of the Mitotic Proteome by SEC-SWATH-MS. Cell Syst. 2020;10(2):133-55.e6.
17. Fossati A, Li C, Uliana F, Wendt F, Frommelt F, Sykacek P, et al. PCprophet: a framework for protein complex prediction and differential analysis using proteomic data. Nature Methods. 2021;18(5):520-7.
18. Savitski MM, Reinhard FB, Franken H, Werner T, Savitski MF, Eberhard D, et al. Tracking cancer drugs in living cells by thermal profiling of the proteome. Science. 2014;346(6205):1255784.
19. Zhu Y, Guo T, Sze SK. Elucidating structural dynamics of integral membrane proteins on native cell surface by hydroxyl radical footprinting and nano LC-MS/MS. Methods Mol Biol. 2011;790:287-303.
20. Stalder L, Banaei-Esfahani A, Ciuffa R, Payne JL, Aebersold R. SWATH-MS co-expression profiles reveal paralogue interference in protein complex evolution. bioRxiv. 2020:2020.09.08.287334.