Stellar MS是赛默飞公司在今年ASMS会议上推出的最新质谱仪。Matthias Mann组率先测试了这台仪器,并于BioRxiv展示了评估结果:https://www.biorxiv.org/content/10.1101/2024.06.02.597029v2
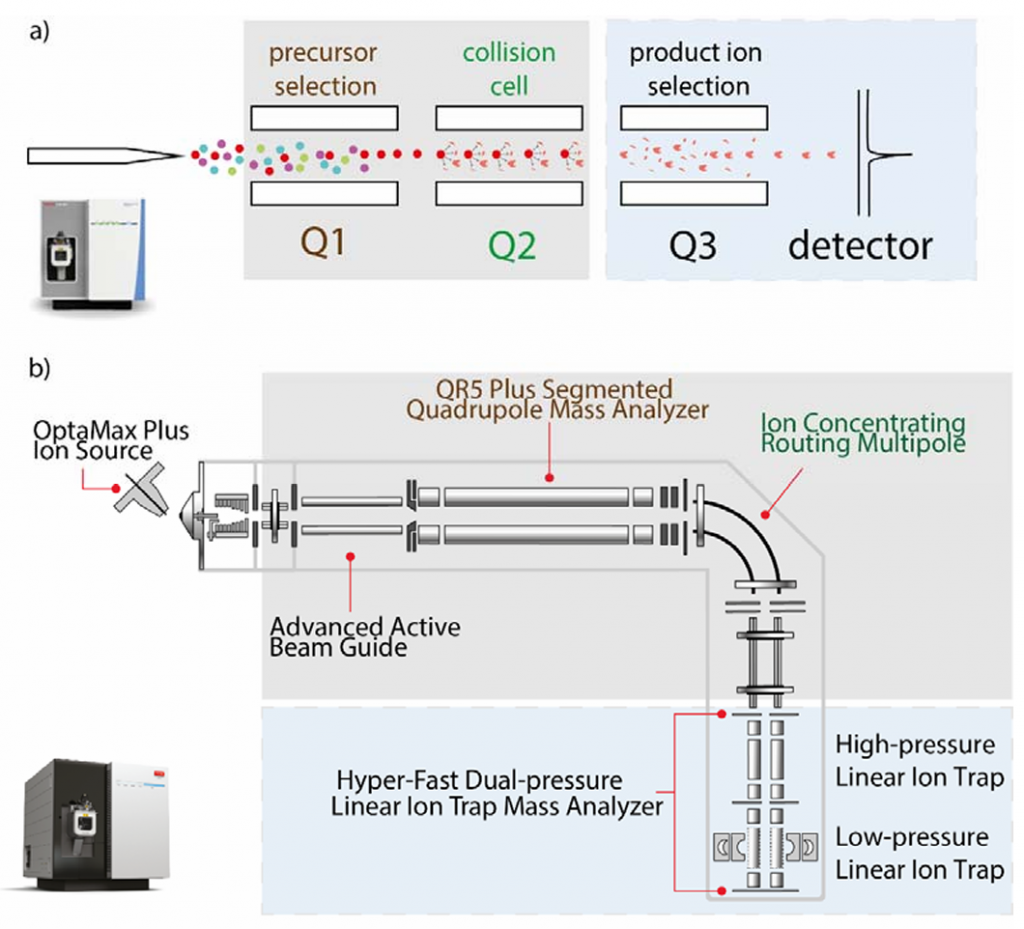
a, 传统三重四级杆质谱(Thermo TSQ Altis Plus);b, Stellar MS.
Stellar MS使用了自家TSQ Altis Plus的机架、真空室、涡轮泵和四极质谱过滤器,以及Orbitrap Ascend Tribrid的离子漏斗和双室线性离子阱。这样的融合让Stellar不仅保留了相对成熟的结构设计、而且允许进行PRM-MS分析而非单纯的SRM-MS,这明显更兼容现今愈加复杂的质谱检测样本。同时,其双离子阱结构允许进行离子积累和碎裂、相较以往的靶向质谱提高了其谱图采集速率(70Hz)。另外,论文中提到其检测器中还采用了新型光电倍增管,这似乎能提高检测器的稳定性和寿命。
为了搭配其主推的靶向检测应用,赛默飞推出了PRM Conductor软件工具以简化分析流程;同时保留了其它格式数据的接口,这也能让Stellar更好跟其它的主推发现应用的质谱(比如Astral等)进行打包售卖。
总之,Stellar以相对简单的仪器架构(可想而知也对应着相对低廉的造价成本)完成了对高级应用(比如文中提到的单细胞多肽检测、高通量临床靶点验证登)的兼容。
生物医学研究中,靶向质谱法往往出现于研究后段、而此时的验证工作往往聚焦到数以十计的标志物。因此,“高通量靶向验证”的概念,笔者认为有待商榷;临床应用里,面对医院源源不断的病患样本,Stellar或许倒能发挥其快速、便捷的优势。赛默飞用Stellar,会不会是要为其进军临床质谱占个山头,我们这几年就会知道。