中文标题:质谱谱图库 Transition available below
A spectral library refers to the collection of existing mass spectrometry (MS)-based peptide and protein information from the analytes, including the precursor and fragment ions m/z, their relative intensity, and standardized retention time (RT) (1). They are essential for analyzing state-of-the-art DIA-MS data, despite with emerging de novo strategies.
Spectral libraries can be generated in three main ways. The most common one is experimentally generated by combining the results of multiple fractions of DDA or DIA analyses from the analyte of interest (2, 3). When an independent experimental library is unavailable, public spectral databases, such as DIA pan human library (DPHL) (4) may be considered. An alternative method, which does not require an external source, employs a library-free, whereas spectrum-centric search (akin to DDA-MS data search) of the DIA-MS data that you want to analyze, and takes the initial identification results as internal library (5, 6), whcih will be used for a second round of more stringent search. While the experimental library provides comprehensive spectral information, the internal library preserves the inherent characteristics of RTs and fragmentation patterns for higher confidence of detection (7). Finally, an emerging field generats an in silico predicted libraries, using deep learning technologies and trained with previously generated MS/MS spectral data (8, 9). This method saves the time and cost required for an experimental library generation, minimizes technical variations from experiments, and can include rare peptides that are otherwise hardly covered by the experiments. As the generation of an internal library or a predicted library does not require external spectral information, many studies regard them as library-free approaches in a broad sense (10-13).
The cutting edge AI technologies have enabled more robust generation of predicted libraries, which in many cases have outperformed internal or experimental libraries (please check AlphaPeptDeep as an excellent example) (14), and shed light in the MS analysis of advanced topics such as immunopeptidomics (15) and single cell biology (16).
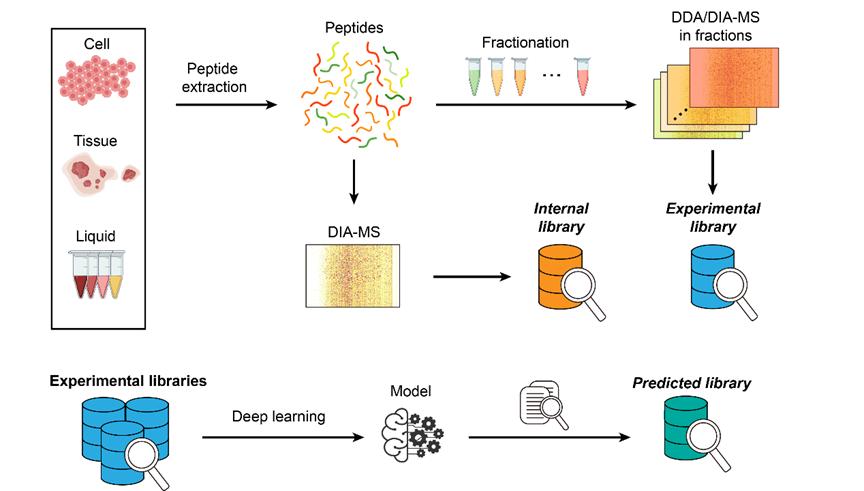
中文翻译
质谱谱图库指的是现有的基于质谱的肽和蛋白质信息的集合,包括感兴趣的分析物的前体离子和碎片离子的m/z值、相对丰度以及标准化的保留时间(1)。尽管最近的“从头算”等不需要谱图库的策略开始兴起,但在其余大多数情况下,库对于分析质谱数据仍然是必不可少的。
质谱库主要可以通过三种方式生成。最常见的一种是实验库,基于感兴趣的分析物的前期实验结果生成(2)(3)。当没有可用的独立实验库时则可以考虑使用公共的质谱数据库,例如DIA pan human library(DPHL)(4)。第二种方式叫做内部库,不需要外部来源、而是先采用类似于DDA-MS数据分析的基于fasta文件的搜索模式对想要分析的DIA-MS数据进行预搜索(5,6),并将初步结果视作内部库用于第二轮更严格的搜索。相比较而言,虽然实验库能提供更大量的肽信息,但内部库由于保留了保留时间和裂解模式等该次质谱实验的特性,从而可以在检测的可靠性上更胜一筹(7)。最后,最近新兴的领域叫做预测库,基于深度学习等计算科学技术、基于给出的多肽序列直接预测其各项信息以生成库(8,9)。这种方法节省了生成实验库所需的时间和成本,减少了实验中的技术差异,并可以涵盖难以通过实验覆盖的稀有肽。由于生成内部库或预测库不需要外部光谱信息,许多研究将其视为广义上的“无库”法(10-13)。
前沿的AI技术正使得预测库的生成更可靠、更精确、而且在许多情况下结果比内部库或实验库更好,新近流行的AlphaPeptDeep方法就是个好例子(14);预测库方法也为更剪短的质谱分析课题,如免疫肽组学(15)和单细胞生物学(16)等等提供了新的思路。
References
1. Schubert OT, Gillet LC, Collins BC, Navarro P, Rosenberger G, Wolski WE, et al. Building high-quality assay libraries for targeted analysis of SWATH MS data. Nat Protoc. 2015;10(3):426-41.
2. Röst HL, Rosenberger G, Navarro P, Gillet L, Miladinović SM, Schubert OT, et al. OpenSWATH enables automated, targeted analysis of data-independent acquisition MS data. Nat Biotechnol. 2014;32(3):219-23.
3. Searle BC, Pino LK, Egertson JD, Ting YS, Lawrence RT, MacLean BX, et al. Chromatogram libraries improve peptide detection and quantification by data independent acquisition mass spectrometry. Nat Commun. 2018;9(1):5128.
4. Zhu T, Zhu Y, Xuan Y, Gao H, Cai X, Piersma SR, et al. DPHL: A DIA Pan-human Protein Mass Spectrometry Library for Robust Biomarker Discovery. Genomics, Proteomics & Bioinformatics. 2020;18(2):104-19.
5. Li Y, Zhong CQ, Xu X, Cai S, Wu X, Zhang Y, et al. Group-DIA: analyzing multiple data-independent acquisition mass spectrometry data files. Nat Methods. 2015;12(12):1105-6.
6. Tsou CC, Avtonomov D, Larsen B, Tucholska M, Choi H, Gingras AC, et al. DIA-Umpire: comprehensive computational framework for data-independent acquisition proteomics. Nat Methods. 2015;12(3):258-64, 7 p following 64.
7. Zhong C-Q, Wu R, Chen X, Wu S, Shuai J, Han J. Systematic Assessment of the Effect of Internal Library in Targeted Analysis of SWATH-MS. Journal of Proteome Research. 2020;19(1):477-92.
8. Gessulat S, Schmidt T, Zolg DP, Samaras P, Schnatbaum K, Zerweck J, et al. Prosit: proteome-wide prediction of peptide tandem mass spectra by deep learning. Nat Methods. 2019;16(6):509-18.
9. Yang Y, Liu X, Shen C, Lin Y, Yang P, Qiao L. In silico spectral libraries by deep learning facilitate data-independent acquisition proteomics. Nat Commun. 2020;11(1):146.
10. Sinitcyn P, Hamzeiy H, Salinas Soto F, Itzhak D, McCarthy F, Wichmann C, et al. MaxDIA enables library-based and library-free data-independent acquisition proteomics. Nat Biotechnol. 2021.
11. Zhang F, Ge W, Ruan G, Cai X, Guo T. Data-Independent Acquisition Mass Spectrometry-Based Proteomics and Software Tools: A Glimpse in 2020. Proteomics. 2020:e1900276.
12. Demichev V, Messner CB, Vernardis SI, Lilley KS, Ralser M. DIA-NN: neural networks and interference correction enable deep proteome coverage in high throughput. Nat Methods. 2020;17(1):41-4.
13. Ting YS, Egertson JD, Bollinger JG, Searle BC, Payne SH, Noble WS, et al. PECAN: library-free peptide detection for data-independent acquisition tandem mass spectrometry data. Nat Methods. 2017;14(9):903-8.
14. Zeng W-F, Zhou X-X, Willems S, Ammar C, Wahle M, Bludau I, et al. AlphaPeptDeep: a modular deep learning framework to predict peptide properties for proteomics. Nature Communications. 2022;13(1):7238.
15. Wahle M, Thielert M, Zwiebel M, Skowronek P, Zeng W-F, Mann M. IMBAS-MS Discovers Organ-Specific HLA Peptide Patterns in Plasma. Molecular & Cellular Proteomics. 2024;23(1):100689.
16. Thielert M, Itang ECM, Ammar C, Rosenberger FA, Bludau I, Schweizer L, et al. Robust dimethyl‐based multiplex‐DIA doubles single‐cell proteome depth via a reference channel. Molecular Systems Biology. 2023;19(9):e11503.